Agentic AI in Biomedical Research: A New Era of Intelligent Collaboration
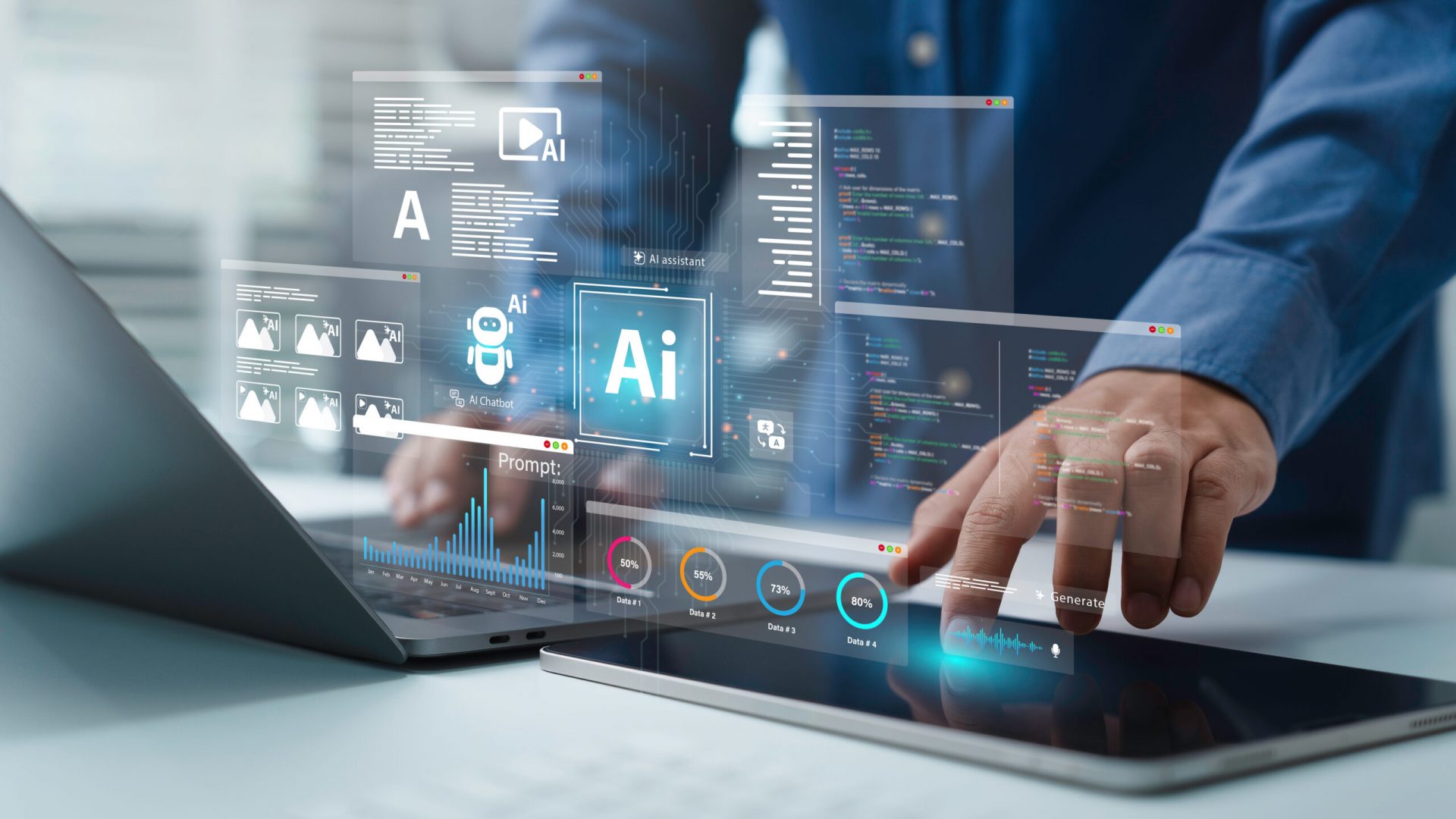
- Apr 24, 2025
- 7 Min. Read
Artificial intelligence has reached a pivotal moment, especially in biomedical research. Intelligent systems that act autonomously and coordinate seamlessly hold the potential to accelerate discoveries, enhance patient outcomes, and streamline how data is gathered and interpreted. At the center of this promise is Agentic AI—technology capable of operating with minimal human oversight and of supporting complex networks of interacting agents. From drug discovery to device surveillance, Agentic AI can reshape research and practice in ways once thought out of reach.
The Challenge: Data Overload and Fragmentation
Biomedical research utilizes diverse data sources, spanning genomics, clinical trials, imaging repositories, and electronic health records. Datasets often sit in isolation, disconnected each other. Researchers spend considerable time locating, harmonizing, and structuring disparate information, while regulators must sift through these troves to find signals about safety and effectiveness. This fragmentation burdens the entire ecosystem. Clinicians may miss vital insights, and regulators face delays in detecting problems with new therapies.
Faced with this complexity, many healthcare and research professionals experience burnout. The administrative work of collating and analyzing data—and ensuring regulatory compliance—can overwhelm even the most capable teams. Although conventional AI solutions have eased these burdens in limited ways, the growing scale of biomedical data demands a more coordinated strategy.
Agentic AI: A Transformative Force
Agentic AI responds to these issues by leveraging multi-agentic systems: networks of autonomous AI “agents” that can share information, assign tasks, and adapt in real time. While many AI solutions focus on narrow tasks, Agentic AI aims to support the entire pipeline—from designing research questions to identifying critical gaps in current knowledge.
An example involves how these agents might spark new avenues of inquiry in drug discovery. One agent could propose research questions by scanning published literature, clinical trial data, and emerging real-world evidence. Another agent could assess gaps and compare them with known priorities—such as rare diseases or unmet therapeutic needs—then rank these questions by potential impact. Researchers would receive a curated list of inquiries backed by an audit trail of data sources. This seamless interplay of agents accelerates project design, cutting months of manual information-gathering to days or even hours.
A Cycle of Inquiry and Validation
One of the most powerful attributes of multi-agentic AI is its capacity to iterate. After new research questions are formulated, another agent can evaluate current data sets for feasibility. If gaps persist, the system flags additional data to collect or prompts new experiments. Once researchers begin their studies, yet another agent tracks progress in real time, compiling findings and adjusting priorities as fresh insights emerge. This cyclical process can repeat at scale, enabling large volumes of concurrent studies—each guided by AI agents skilled in data synthesis and gap analysis.
Mass analysis plays a crucial role here. By running many inquiries in parallel, Agentic AI can unearth trends and connections that human teams might overlook. Regulators could review aggregated findings on a shared platform, ensuring that new therapies or devices meet safety and efficacy standards more efficiently. Clinicians could tune treatments based on the most recent data, and patient advocacy groups could see how research evolves in near real-time.
From Bench to Real-World Evidence
Agentic AI does not end with early-stage discovery. Once a therapy or device reaches clinical use, it requires active surveillance. Multi-agentic systems can integrate safety reports, electronic health records, and patient feedback to pinpoint possible issues. If a previously unknown side effect surfaces, the agents alert the appropriate parties, triggering deeper investigation. This continuous flow of information protects patient welfare and guides long-term research decisions.
Adherence to FAIR (findable, accessible, interoperable, and reusable) principles ensures that data stays transparent and well organized. Researchers can collaborate on shared platforms where each agent interacts with large datasets in a governed and traceable manner. Privacy-preserving methods keep patient information secure while allowing for robust analysis.
Collaboration, Speed, and Impact
The true potential of Agentic AI emerges when it unites researchers, clinicians, regulators, and patients around a common framework. Through the power of cloud computing, advanced analytics, and cross-disciplinary knowledge, these systems can tackle research challenges once deemed too large or convoluted. By automating routine tasks and providing timely insights, they free human teams to focus on strategy, creativity, and clinical acumen.
This synergy fosters trust. Regulators can see precisely how AI-driven evaluations were conducted, clinicians can observe real-time data curation, and patients gain clarity about how emerging therapies are scrutinized for safety. Such openness is crucial for building the confidence that underpins wide-scale adoption of next-generation biomedical solutions.
Navigating Ethical Imperatives
With great promise comes responsibility. The application of Agentic AI in biomedical research must address pressing ethical concerns, including bias detection, data governance, and thorough validation of each agent’s outputs. Human oversight remains essential at pivotal moments, and agentic systems should incorporate feedback loops that measure performance against clinical outcomes. A commitment to transparency and accountability at every step ensures that these technologies advance public health rather than undermine it.
Call to Action: Driving Innovation Responsibly
As data volumes rise and the demands on biomedical research grow more urgent, multi-agentic approaches offer not only efficiency but also a chance to transform how science is done. Agentic systems can propose research questions, highlight unmet needs, launch multiple studies simultaneously, and continually update stakeholders with findings. These capabilities offer a pathway to accelerate discovery while preserving rigor and equity.
At ICA, we believe that the future of healthcare depends on thoughtful, cross-disciplinary methods that meld technology and humanity. Our mission is to connect breakthroughs in AI with the real-world needs of patients, clinicians, and regulators. By fostering collaboration at every level, we stand ready to guide stakeholders as they implement, refine, and oversee the next generation of agentic systems.
Agentic AI represents more than an evolution in computational power. It is a step toward continuous, intelligent collaboration that enriches biomedical research while safeguarding patient wellbeing. This future is within reach—a future in which science, technology, and conscientious practice converge to create healthier lives for all.