Personalized Medicine Meets AI: Unlocking the Power of Big Data
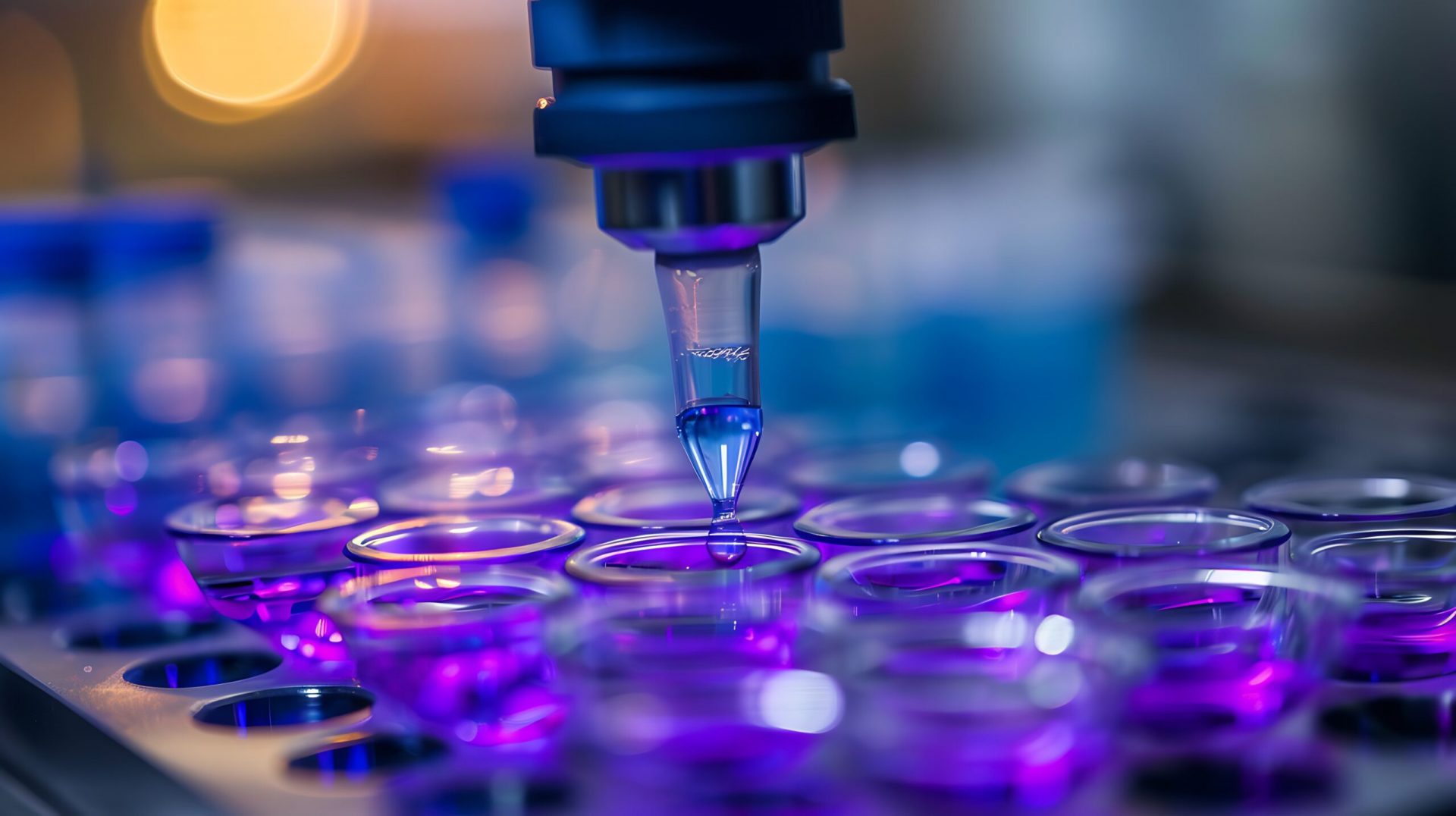
- Feb 5, 2025
- 5 Min. Read
What if your treatment plan was as unique as your DNA and lifestyle? Personalized medicine promises to make that a reality, and AI is leading the charge. Personalized medicine is a medical approach that tailors treatments and healthcare strategies to the individual characteristics of each patient. Rather than relying on a one-size-fits-all approach, personalized medicine takes into account a patient’s genetic makeup, lifestyle, and environment to design more precise therapies and prevention strategies. The goal is to improve treatment efficacy, reduce side effects, and enhance overall health outcomes by identifying the most effective healthcare plan for each patient. In practice, personalized medicine often involves genetic testing, advanced imaging, biomarker analysis, and other health data analyses to guide decisions about patient care.
As this vision becomes reality, the challenge lies in unlocking the full potential of increasingly varied biomedical data. Real-world data, genomic information, and unstructured clinical notes hold transformative insights, but only when harnessed effectively. Successfully navigating this complexity requires not just advanced AI tools, but a strategic understanding of how to integrate cutting-edge technologies into clinical, research, and regulatory workflows. Those who can bridge these domains stand at the forefront of delivering truly individualized care.
Challenges with “Big” Data
Personalized medicine represents a transformative shift in healthcare, requiring the effective use of varied, high-dimensional data. From real-world evidence and molecular data to unstructured clinical notes, these datasets hold the promise of more precise treatments but also present significant challenges.
High-dimensional datasets often contain vast amounts of information, yet they can produce sparse data distributions that make statistical patterns harder to detect. Models trained on such data risk capturing noise instead of meaningful insights, reducing their reliability and generalizability. Additionally, managing and analyzing these large datasets requires substantial computational resources, which can limit scalability and hinder widespread adoption. These challenges necessitate innovative approaches to unlock the full potential of varied biomedical data in personalized medicine.
Promising AI Implications for “Big” Data
Equally burgeoning and transformative is the deployment of Artificial Intelligence (AI) and machine learning approaches (ML) on healthcare data to inform personalized treatment approaches. AI/ML-directed principal components analysis (PCA), t-distributed stochastic neighbor embedding (t-SNE) and autoencoders have shown promise in reducing dimensionality while also identifying and retaining informative data features; and specific feature selection algorithms, such as recursive feature elimination (RFE), aid in isolating relevant variables. Advanced deep learning models, including convolutional neural networks (CNNs) for imaging data, enable the direct navigation of high-dimensional inputs by learning hierarchical feature representations. Ensemble methods, such as random forests and gradient boosting machines, have demonstrated robust performance and resistance to model overfitting in complex datasets.
AI/ML can also assist in detecting anomalies and bias within large datasets. AI algorithms, including clustering and one-class support vector machines (SVM), excel at identifying outliers; it’s also quite reasonable to expect that such techniques could be modified to identify common biases present in subsets of high-dimensional data (e.g. selection bias). Additionally, Explainable AI (XAI) techniques like Shapley additive explanations (SHAP) and local interpretable model-agnostic explanations (LIME) can improve interpretability of results by highlighting feature importance. AI/ML tools also have the potential to adapt imputation models and robust estimators to reduce the impact of missing data.
Applications of AI in Personalized Medicine
AI is poised to play a pivotal role in advancing personalized medicine. By integrating and analyzing varied biomedical data—including electronic health record data, molecular data, and patient-generated health information—AI is transforming how we understand and treat disease. As molecular data become increasingly affordable to capture, AI will be especially helpful in performing multi-omics analyses, which link high-dimensional datasets, and are unlocking insights into complex disease mechanisms and potential treatments.
AI is also making strides in drug discovery and development. By analyzing patterns in complex datasets, AI tools can identify novel drug targets, predict drug responses, and streamline clinical trials. The integration of real-world data into these processes enables more accurate forecasts of disease risk, progression, and treatment outcomes.
Additionally, AI-driven diagnostic tools are reducing errors by synthesizing varied data sources. For instance, combining electronic health records (EHR), imaging data, and genomics allows for more accurate and timely diagnoses. AI’s role extends to telemedicine platforms, enabling remote diagnostics and personalized treatment recommendations, which improve accessibility and reduce healthcare costs.
Federated learning, a machine learning approach that trains models collaboratively without sharing raw data, is emerging as a solution to privacy concerns. By enabling decentralized training across datasets, federated learning preserves patient confidentiality while advancing research. Similarly, AI-generated synthetic datasets provide a promising avenue for algorithm training without compromising sensitive health information.
Challenges with AI in Personalized Medicine
While AI holds tremendous promise, its integration into personalized medicine faces distinct challenges. Data privacy, security and ethical considerations are at the top of the list; safeguarding sensitive health information will be of paramount importance. Moreover, prioritizing informed consent, establishing accountability and liability for AI-directed systems, careful monitoring of autonomy and decision-making, minimization of bias in AI models, and ensuring accessibility are all challenges that will require deep evaluation.
Interoperability with existing healthcare systems remains another significant hurdle. AI tools must align with established workflows to gain clinical buy-in and ensure seamless implementation. Regulatory clarity is also essential to build trust and support widespread adoption. Current regulatory frameworks often struggle to accommodate AI systems that continuously learn and adapt, leaving gaps in oversight. Addressing these challenges will require collaboration among data scientists, clinicians, and regulators to ensure that AI applications in healthcare are safe, equitable, and effective.
A Look to the Future & the Regulatory Landscape
The future of personalized medicine lies at the intersection of AI and biomedical data. By addressing the challenges posed by varied datasets, AI can unlock transformative insights and enable more precise, effective healthcare and improve patient outcomes. By addressing challenges and fostering interdisciplinary collaboration, AI tools can unlock the full potential of precision healthcare. With an eye on the future, the regulatory landscape seems to present the largest unknowns for the healthcare industry. The integration of AI into personalized medicine will require a robust regulatory framework to ensure safety, efficacy, and ethical use. Governments and health organizations worldwide are working to establish guidelines that balance innovation with patient protection. The U.S. Food and Drug Administration (FDA) has introduced the Artificial Intelligence/Machine Learning-Based Software as a Medical Device (SaMD) Action Plan. This framework emphasizes transparency, real-world performance monitoring, and iterative updates to AI algorithms; the EU has proposed similar legislation (AI Act). Oversight challenges will persist though, since typical software regulations may not apply to AI systems that continuously learn and adapt; further, standardized protocols and data exchange formats have yet to be agreed upon and adopted, making integration of AI tools into existing health systems difficult.
Advancing personalized medicine demands more than technology; it requires leadership in navigating the intersection of clinical practice, research, and regulatory landscapes. The integration of AI tools into healthcare workflows, combined with a commitment to establishing shared standards and fostering interdisciplinary collaboration, will shape the future of medicine. Those who can unify these varied elements to unlock the potential of AI are poised to drive meaningful progress, ensuring that this era of transformation delivers on its promise of better outcomes for all.